“Prediction is very difficult, especially about the future.”
—Niels Bohr, Danish physicist (1885 – 1962)
Niels Bohr is not the only one who has had trouble predicting the future. This article questions the ability of long-term climate models to accurately predict the climate. By extension, this article questions the wisdom of implementing expensive and disruptive policies aimed at combating climate change, such as corporate average fuel economy (CAFE) standards, carbon cap and trade plans, the ban on incandescent light bulbs, and the Environmental Protection Agency’s (EPA) proposed tax and regulation of carbon dioxide under the 1970 Clean Air Act.
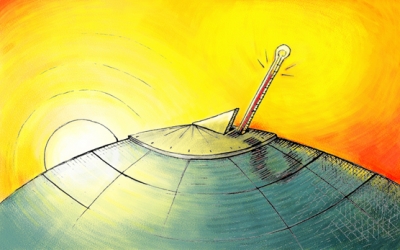
Illustration by Barbara Kelley
Climate models are composed of five basic components:
- Model “inputs,” such as atmospheric carbon dioxide levels, atmospheric particulate levels, and solar radiation from, say, 1700 to 2010. We believe these “inputs” are driving the model “outputs.”
- Model “outputs,” such as land surface temperatures from 1700 to 2010.
- The relationships between key inputs and outputs. By studying the historical inputs, such as carbon dioxide, and historical outputs, such as temperatures, some mathematical relationship between the two can be established. This relationship causes us to believe we know how the inputs are affecting the outputs during the historical period. We assume that this relationship will hold into the future.
- A trend of the inputs. We want our model to forecast the future, not the past, so we need to predict the future levels of the inputs. Perhaps carbon dioxide levels are trending up and, at current rates of growth, will hit 400 parts per million in the atmosphere by 2015.
- The desired answer. Given the trends in the input quantities and the mathematical relationships between the inputs and outputs, what are the values of the outputs (such as surface temperatures) in a certain place (such as Hawaii) at a certain time (such as 2015)? This is what the model produces and this prediction cannot be substantiated until 2015.
Let’s consider each of these components. How are historical temperatures known, other than through actual thermometer readings? Of course, no one has been keeping good thermometer readings of Hawaii’s temperature since 1700. We can, however, get historical temperatures from tree rings, which show cold and warm growing years. How are historical carbon dioxide levels known? Ice cores can provide this. How about old solar radiation levels? Surprisingly, fossilized seashells can provide this information. While not perfect, historical model input and output readings appear to be perfectly adequate.
How can we determine if a model truly has predictive ability?
Climate models start showing their weaknesses in the physical relationships they capture—or don’t capture. Consider clouds. Clouds should definitely be included in climate models because, as we know, clouds both reflect incoming and trap outgoing radiation. Clouds thus play a critically important role in the earth’s climate. If the sky is cloudy on a winter night, then the air will be warmer than on a night with clear skies. If the world really heats up, will there be more or less cloud cover? Does the climate drive clouds or do clouds drive the climate? It turns out that scientists don’t know.
Here’s what Henrik Svensmark, director of the Center for Sun-Climate Research at the Danish National Space Center in Copenhagen, wrote:
All we know about the effect of [carbon dioxide] is really based on climate models that predict how climate should be in 50 to 100 years, and these climate models cannot actually model clouds at all, so they are really poor. When you look at them, the models are off by many hundreds percent. It’s a well-known fact that clouds are the major uncertainty in any climate model. So the tools that we are using to make these predictions are not actually very good.
The Pew Center on Global Climate Change adds:
Uncertainty remains about how particular aspects of climate change will play out in the future, such as changes in cloud cover or the timing or magnitude of droughts and floods.
Even the Intergovernmental Panel on Climate Change (IPCC) concurs, saying:
Significant uncertainties, in particular, are associated with the representation of clouds, and in the resulting cloud responses to climate change.
It’s not that climate scientists have ignored clouds due to an oversight; it’s that scientists don’t understand how clouds are formed. That’s where Svensmark comes in. His research suggests that clouds are formed via deep space cosmic rays hitting the earth. These cosmic rays are, in turn, affected by the amount of radiant energy put out by our sun. The sun’s energy blocks these cosmic rays. When the sun’s energy output is high, as it has been recently, there are fewer cosmic rays hitting earth, fewer clouds being formed, and the earth heats up. This large effect from cloud creation overshadows the minor effect from additional solar radiation hitting our planet.
This is a hot topic and just last month CERN, the European Organization for Nuclear Research, released some preliminary results of an experiment designed to look into exactly this method of cloud formation. Are these cosmic ray/cloud relationships included in climate models? How could they be if the relationships are not fully understood?
Scientists don’t understand how clouds are formed.
Other questions to ask about climate models are: how accurately do they reflect reality and how well do they predict the future? In order to answer these questions, we have to validate the model. There are really three components to model validation: (1) calibration, (2) holdout testing, and (3) long-term testing.
Calibration means building the model and having it provide us with data we already know. We do that by comparing the modeled values—those that come out of the model—with actual historical data. Climate models do a good job in this respect. According to the Pew Center on Global Climate Change, “Most of the current models do a reasonable job of simulating past climate variability on time scales of decades to centuries…”
Holdout testing, or hindcasting, is the next step. We hold out, or hide, some of our historical data, and then rerun the model without those data. If the model’s results are close to the actual, held-out values, then we can start to believe that the model reflects reality with some degree of accuracy.
As good as holdout testing is, it isn’t perfect. Ask yourself the following question: What’s the chance of a meltdown in the residential housing market? In 2005, you might have assigned a low probability. Now that you’ve lived through the subprime fiasco and the housing market collapse, you will, whether consciously or subconsciously, assign higher probabilities to such a “rare” event. Whether modelers are honest with themselves or not, it is impossible to ignore what we know has already happened, and if a model fails to replicate known events, it will be tweaked until it does.
Just like statisticians, we modelers have ways to fool others and ourselves. Here are five ways to be bamboozled by deceptive models:
- I can build a “model” of the stock market with a simple lookup table. What was the Dow Jones Industrial Average (DJIA) on May 31, 2011? It was 12,290. Check. What was it on July 19, 2011? It was 12,572. Check. You can query the model for any historical period and the model will reference the lookup table, providing an answer that will be spot on. Guaranteed. It will also have zero predictive value.
- I can build a model that shows “relationships” between the DJIA and thousands of irrelevant variables, such as sports scores, movie attendance, murder rates, hospital infections, air temperatures, etc., using a technique called multiple linear regression. I guarantee that some relationships, albeit spurious, will be “discovered.” This model would be finely calibrated but it would have zero or limited predictive ability.
- I could trend historical DJIA data with a polynomial that is well behaved within the historical period but shoots out of control for future predictions, as capricious polynomials often do. This model, also, would be finely calibrated but it would have limited predictive ability.
- The trends in my model could ignore known factors and patterns. For instance, trend daily Christmas tree sales from mid-November through mid-December and you could predict, quite incorrectly, that Christmas tree sales will be strong through the spring.
- I could build a large number of models or have each model run a large number of scenarios. Then, at some point in the future, I could unveil the one that happened to get lucky, designate it the winner, and claim victory.
How can we determine if a model truly has predictive ability? There’s really only one way. We finish it, lock it down, and put it away like a good bottle of Bordeaux. The reason we lock it down is that each time model inputs are altered, even slightly, the model results might change dramatically. Each time we tweak a variable, we are effectively giving the model a “peak ahead” to events that have already happened and the clock needs to start anew. As economist David Friedman points out, “In order to test the predictions of a model you need to do it against actual predictions—information that didn't go into building the model.”
Just like statisticians, we modelers have ways to fool others and ourselves.
It is inevitable that our models will evolve as computing power, theory, and climate measurements progress. Once the models are stable, however, they should be stored for a length of time sufficient to “age” them and test their predictive ability. Climate models have had some success in this regard, such as NASA’s Goddard Institute for Space Studies’ model of 1988, in which actual measured temperatures matched reasonably well the model’s middle scenario for a decade and a half. But successes like this are just baby steps.
How long should climate models be put aside to test their predictive value? If we are trying to predict the climate for the next 100 years, the models should be put away for at least a few decades because it will always be easier for them to nail down the 10-year forecast than the 100-year forecast. That’s due to the fact that there’s less uncertainty in the near term than in the long term.
This uncertainty, or variability, in the weather is another reason to put the models aside for longer. Consider the historical snowfall chart for Donner Summit in the Sierra Nevada mountain range. There doesn’t seem to be any pattern; the data seems to be truly random, with the snowfall of one year having little relationship to the snowfall of another.
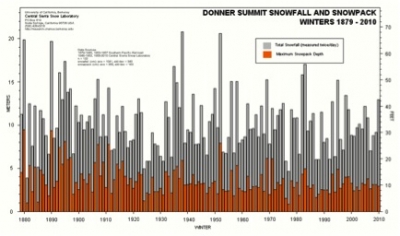
Data like the above make it hard to discern the underlying trend in the climate. Do two warm years mean the earth is warming or are those just a sign of the randomness of the weather? This requires us to test our climate models for longer periods to be sure we are picking up true climate trends rather than just “noise.”
Consider the Intergovernmental Panel on Climate Change’s following claim that climate models are accurate. “There is considerable confidence that climate models provide credible quantitative estimates of future climate change, particularly at continental scales and above. This confidence comes from the foundation of the models in accepted physical principles and from their ability to reproduce observed features of current climate and past climate changes.”
The IPCC is saying that these models are accurate because they model known physical principles properly and have the ability to replicate past events. What if there are unknown physical principles that can’t be modeled because they aren’t known, like the cosmic rays and cloud formation? And the ability to replicate past events covers only the first or, perhaps, the first and second, of the three requirements for validating models.
That climate models need to prove their predictive ability in the long-term is a given. The question is: What do we do in the meantime? Some, like climate change blogger Coby Beck, say we need to act now: “It is only long-term predictions that need the passage of time to prove or disprove them, but we don't have that time at our disposal.”
Other people, including myself, think that if long-term climate models are slated to dictate trillion-dollar decisions affecting the world economy, they must prove their accuracy. With such a fundamental omission as cloud formation, climate models may be more misleading than helpful. Predicting the future of something as complicated as the earth’s climate is indeed difficult with inadequate and unsubstantiated climate models.
Outstanding article. I am sending links to my friends.
---Owen Hughes
Charles Hooper's article, 'Predicting Our Demise,' has a fundamental flaw. He states, 'How can we determine if a model truly has predictive ability. There's really only one way. We finish it, lock it down, and put it away like a good bottle of Bordeaux. The reason we lock it down is that each time model inputs are altered, even slightly, the model results might change dramatically.' Unfortunately, 'locking it down' does nothing towards reducing the uncertainty that we deal with when making decisions about the future. And it is not very useful as a way to compare models.
---Spencer Star